Get in Touch
- Reach out to Another Life Prod for all your SMSF needs.
- Address: [4576 Harper Street Madisonville, KY 42431]
- Phone: [270-452-9775]
- Email: [[email protected]]
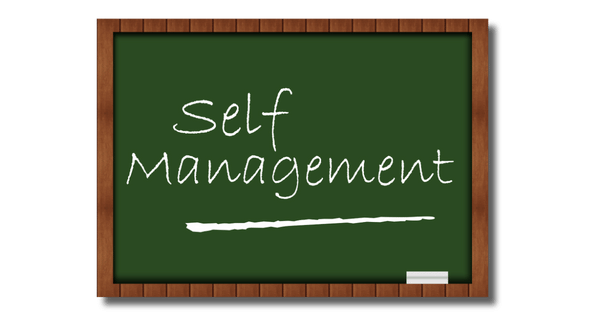
Another Life Prod specializes in Self Managed Super Fund (SMSF) setup and management.
Explore our comprehensive services to secure your financial future.
Setting up a Self Managed Super Fund (SMSF) involves several expenses, including legal and administrative fees, initial contributions, and ongoing management fees. The total SMSF setup costs can vary depending on the complexity of your fund and the services you require. It’s essential to budget for these expenses and seek professional guidance to ensure a smooth and cost-effective SMSF establishment process.
Experience hassle-free SMSF setup online with Another Life Prod. Our user-friendly platform makes it easy to establish your self-managed super fund quickly and efficiently. Say goodbye to complex paperwork and lengthy processes – our online system streamlines the setup, ensuring a seamless experience. Take control of your financial future with our convenient online SMSF setup services.
SMSF management refers to the ongoing administration and oversight of a Self Managed Super Fund (SMSF). This crucial task involves monitoring investments, ensuring compliance with regulatory requirements, and maintaining accurate financial records. Effective SMSF management ensures the fund’s long-term financial stability and helps members achieve their retirement goals through strategic decision-making and prudent financial management.
Our SMSF management services offer expert guidance and seamless administration for your Self Managed Super Fund (SMSF). With transparent fees and a dedicated team of professionals, we ensure your SMSF is compliant and optimized for growth. Trust us to handle the complexities of SMSF management, allowing you to focus on your financial goals with confidence.